3
Genetic Test Assessment
Diagnostic and predictive tests used in medical settings have potential benefits and harms, and genetic tests are no exception. Some genetic tests are used in circumstances that, although not unique to genetic tests, offer particular challenges in evaluating the balance of benefits and harms. For example, the condition of interest might be uncommon or rare; interventions might be limited; different clinical outcomes might be preferred, depending on the stakeholder; tests might not be rigorously reviewed until after their clinical introduction; and there might be inadequate and conflicting evidence and guidance regarding a test’s use. Genetic tests also present complex ethical, legal, and social implications (ELSI) that need to be examined. Therefore, methods have been developed to guide stakeholders (patients, clinicians, health care system policy makers, payers, and public health officials) in the assessment of tests, including genetic tests, in a broad array of clinical settings. Some of the methods have been developed specifically for the assessment of genetic tests. The terms used by different authorities to describe the methods are not always consistent, and the process might have been developed for different purposes. Therefore, in this report, the committee uses the terms method and process to refer broadly to the various systems without specifying their intended applications.
This chapter reviews and compares available methods that have been proposed for reviewing evidence and making decisions about using tests, including several methods designed specifically for genetic tests, and it offers a synthesis that maps clinically relevant outcomes to a hierarchic evidence structure.
EVALUATING GENETIC TESTS
Ideally, the clinical use of a genetic test should be preceded by studies to confirm that it is valid and useful. Two principal measures of validity apply to genetic tests: analytic validity and clinical validity. A third important measure of a genetic test is its clinical utility (NIH, 2016). Those issues are introduced here and discussed in more detail later in this chapter and in Chapter 4.
- The analytic validity (technical test performance) of a genetic test is its ability to test accurately and reliably for the genetic variants of interest in the clinical laboratory in specimens that are representative of the population of interest. Analytic validity
-
includes analytic sensitivity (false-negative results), analytic specificity (false-positive results), within- and between-laboratory precision, and assay robustness (reproducibility among operators, reagent lots, instruments, temperatures, and so on) (Teutsch et al., 2009).
- The clinical validity of a genetic test is its ability to identify or predict accurately and reliably the clinically defined disorder or phenotype of interest. Clinical validity encompasses clinical sensitivity and specificity and predictive values of positive and negative tests that take into account the prevalence of the disorder (Teutsch et al., 2009). Clinical validity might also be expressed as a measure of association, such as a risk ratio or an odds ratio, although such a measure is an incomplete representation of clinical validity.
- The clinical utility of a genetic test is the evidence that it improves clinical outcomes measurably and that it adds value for patient management decision making compared with current management without genetic testing (Teutsch et al., 2009).
In efforts to determine the quality and assess the value of genetic tests, researchers and several public and private organizations have developed methods for evaluating them in clinical settings.
METHODS FOR EVALUATING GENETIC TESTS
The committee began its review by examining a 2011 report from the Department of Health and Human Services’ Agency for Healthcare Research and Quality (AHRQ)1 that addressed many of the issues surrounding genetic testing, including the feasibility of designing a framework for evaluating genetic tests by modifying existing methods, the strengths and limitations of different methods of literature searching to identify evidence, the feasibility of applying existing rating criteria to analytic-validity studies of genetic tests, and gaps in the evidence on sources and contributors of variability that are common to all genetic tests. The report defined evaluation methods, reviewed published methods, identified the specific needs of different stakeholders for evaluation methods, and discussed the feasibility of adapting existing methods to fit a wide array of genetic testing scenarios, such as diagnosis, prognostic evaluation, screening for heritable medical conditions, carrier screening for reproductive purposes, and pharmacogenetics (Sun et al., 2011).
The goal of the AHRQ report was to determine whether it was feasible to offer a comprehensive framework or set of frameworks for evaluating genetic tests. The report (Sun et al., 2011) distinguishes between an evaluation framework and an analytic framework and notes that
an evaluation (or “organizing”) framework for medical test assessment serves the purpose of clarifying the scope of the assessment and the types of evidence necessary for addressing various aspects of test performance and their consequences. Some evaluation frameworks (e.g., the Fryback-Thornbury hierarchy) only provide general conceptual guidance to the evaluators or reviewers. Analytic frameworks (e.g., the frameworks developed by the U.S.
___________________
1 Through its evidence-based centers, AHRQ sponsors the development of evidence reports and technology assessments to assist public and private organizations in improving the quality of health care in the United States.
Preventive Services Task Force [USPSTF] and the Evaluation of Genomic Applications in Practice and Prevention [EGAPP] Working Group) provide additional detail for a set of key questions (e.g., the relevant populations, interventions, comparators, outcomes, time points, and settings . . .).
The AHRQ report evaluated four commonly used methods that cover the principal domains of test evaluation used in the environment of genetic testing: analytic validity, clinical validity, and clinical utility. The four methods, described here chronologically, are the USPSTF method, the Fryback–Thornbury hierarchy (Fryback and Thornbury, 1991), the analytical validity, clinical validity, clinical utility, and associated ethical, legal, and social implications (ACCE) model (Haddow and Palomaki, 2003), and the EGAPP method (Teutsch et al., 2009). The methods are related to different components of a complete decision framework. Of the four, only the USPSTF and EGAPP methods are aimed specifically at making decisions for clinical use. Fryback–Thornbury and ACCE are more general structures for assessing evidence. The committee also reviewed several reports that focused on evaluation frameworks (e.g., Morrison and Boudreau, 2012) and the evaluation process developed by Giacomini and colleagues at McMaster University (Giacomini et al., 2003). The McMaster University evaluation framework was of particular interest to the committee because of the thoroughness of its approach, the richness of its detail, its focus on making coverage decisions for new predictive genetic tests, and its flexibility in applying criteria.
The US Preventive Services Task Force
The USPSTF was established in 1984 to conduct scientific evidence-based reviews on a wide array of preventive services (such as screening, counseling, and preventive medications). It is an independent, volunteer panel of national experts in prevention and evidence-based medicine. Although its methods were developed specifically to inform clinical decisions about preventive interventions in primary care settings, USPSTF was an early innovator in the movement toward more evidence-based practice in general, and its methods have been widely cited and adapted for other clinical domains. All recommendations and supporting evidence reviews are published on the task force’s website and in peer-reviewed journals.2 Since 1998, AHRQ has convened USPSTF and provided continuing scientific, administrative, and dissemination support. Each year, USPSTF provides a report to Congress that identifies critical evidence gaps in research related to clinical preventive services and recommends high-priority subjects that deserve further examination.
USPSTF uses the same framework for evaluating genetic tests as it does for broadly defined preventive services in the primary care setting: screening, counseling, and preventive medications. It examines any available direct evidence from randomized controlled trials (RCTs)3 or roughly equivalent indirect evidence that is guided by a “chain of evidence” constructed within an analytic framework and accompanying key questions (Sawaya et al.,
___________________
2 Available at: https://www.uspreventiveservicestaskforce.org/Page/Name/recommendations (accessed January 31, 2016).
3 RCTs are studies in which people are randomly assigned to two (or more) groups to test a specific drug, treatment, or other intervention. One group (the experimental group) receives the intervention being tested, and the other (the comparison or control group) receives an alternative intervention or no intervention at all. The groups are followed to see how effective the experimental intervention was. Outcomes are measured at specific times, and any difference in response between the groups is assessed statistically.
2007); insufficient or poor-quality direct evidence determines the need for indirect evidence. The primary focus is on evidence that directly or indirectly relates the intervention of interest (such as a medical test) to health benefits and harms that the patient can perceive. ELSI might be considered as they apply to specific topics. And economic costs might be considered but do not have high priority (Morrison and Boudreau, 2012). The USPSTF analytic framework defines which questions must be answered, which types of evidence and information are relevant to the analysis, and by which criteria the evidence will be weighed.
In evaluating evidence, the USPSTF method considers both the following key questions and the overall certainty of the evidence of net benefit of the preventive service in question:
- Do the studies have the appropriate research design to answer the key question(s)?
- To what extent are the existing studies of high quality? (That is, what is the internal validity?)
- To what extent are the results of the studies generalizable to the general US primary care population and situation? (That is, what is the external validity?)
- How many studies that address the key question(s) have been conducted? How large are the studies? (That is, what is the precision of the evidence?)
- How consistent are the results of the studies?
- Are there additional factors that assist us in drawing conclusions (such as the presence or absence of dose–response effects and the fit within a biologic model)?
The overall evidence of net benefit of a preventive service is rated as of “high,” “moderate,” or “low” certainty in light of the extent to which an uninterrupted chain of evidence exists throughout the analytic framework. In this system, conclusions based on high-certainty evidence are unlikely to be strongly affected by the results of future studies, but the magnitude or direction of conclusions regarding an observed effect based on moderate-certainty evidence could change as more information becomes available, and such a change might be large enough to alter the conclusions. Conclusions based on low-certainty evidence are insufficient for assessing effects on health outcomes. USPSTF also synthesizes estimated magnitudes of benefits and harms into an estimate of the magnitude of net benefit. The certainty and magnitude of net benefit are linked to the recommendation, in letter grades (see Table 3-1), about provision of the service in question. USPSTF recommendations are intended to help primary care clinicians and patients to decide together whether a preventive service is right for a given patient’s needs.
TABLE 3-1 USPSTF Letter Grades (since July 2012)
Grade | Definition | Suggestions for Practice |
---|---|---|
A | The USPSTF recommends the service. There is high certainty that the net benefit is substantial. | Offer or provide this service. |
B | The USPSTF recommends the service. There is high certainty that the net benefit is moderate or there is moderate certainty that the net benefit is moderate to substantial. | Offer or provide this service. |
C | The USPSTF recommends selectively offering or providing this service to individual patients based on professional judgment and patient preferences. There is at least moderate certainty that the net benefit is small. | Offer or provide this service for selected patients depending on individual circumstances. |
D | The USPSTF recommends against the service. There is moderate or high certainty that the service has no net benefit or that the harms outweigh the benefits. | Discourage the use of this service. |
I | The USPSTF concludes that the current evidence is insufficient to assess the balance of benefits and harms of the service. Evidence is lacking, of poor quality, or conflicting, and the balance of benefits and harms cannot be determined. | Read the clinical considerations section of the USPSTF Recommendation Statement. If the service is offered, patients should understand the uncertainty about the balance of benefits and harms. |
NOTE: USPSTF = US Preventive Services Task Force.
SOURCE: USPSTF, 2013.
The Fryback–Thornbury Hierarchic Model of Efficacy
The Fryback–Thornbury model, proposed in 1991, provides conceptual guidance for evaluating the efficacy of health technologies at different levels of a hierarchy. It is a widely used general evaluation structure for medical-test assessment and for clarifying the scope of the assessment and the types of evidence necessary for addressing various aspects of test performance and their consequences, including societal effects (Sun et al., 2011; Morrison and Boudreau, 2012). The model describes six levels of efficacy (see Box 3-1) in a hierarchy that the authors recommend be addressed in sequence. The authors underscored the importance of RCTs for tests that have greater risk of harm, greater expense, or wider use. They suggested that decision modeling could be helpful for giving provisional answers or for focusing research efforts on the most important questions. The proposed use of their method was to classify the published evidence on a diagnostic test and describe the conceptual continuum of efficacy (Fryback and Thornbury, 1991).
The ACCE Model
The Centers for Disease Control and Prevention’s (CDC’s) Office of Public Health Genomics established and supported the ACCE Model Project from 2000 to 2004 to develop the first publicly available analytic process for evaluating scientific evidence on emerging genetic tests. ACCE takes its name from the four main criteria or principles used for evaluating a genetic test: analytic validity, clinical validity, clinical utility, and associated ELSI. The ACCE framework has been used in the United States and worldwide for evaluating genetic tests. It was adopted and modified by the Genetic Testing Network in the United Kingdom (Sanderson et al., 2005).
The ACCE process includes collecting, evaluating, interpreting, and reporting categorical evidence on particular genetic tests so that policy makers have access to current and reliable information (Morrison and Boudreau, 2012). The process comprises a standard set of 44 targeted questions (see Table 3-2) that are used to frame each of the major categories. Questions also address the nature of the disorder, the clinical setting, and the type of testing. Economic
considerations are a component of the evaluation of clinical utility. Several additional factors are considered, such as access to downstream remedies or actions, access for vulnerable populations, quality assurance measures, educational materials, and evaluation of program performance.
TABLE 3-2 ACCE Model List of 44 Targeted Questions Aimed at a Comprehensive Review of Genetic Testing
Element | Component | Specific Question |
---|---|---|
Disorder/Setting |
1. What is the specific clinical disorder to be studied? |
|
2. What are the clinical findings defining this disorder? |
||
3. What is the clinical setting in which the test is to be performed? |
||
4. What DNA test(s) are associated with this disorder? |
||
5. Are preliminary screening questions employed? |
||
6. Is it a stand-alone test or is it one of a series of tests? |
||
7. If it is part of a series of screening tests, are all tests performed in all instances (parallel) or are only some tests performed on the basis of other results (series)? |
||
Analytic Validity |
8. Is the test qualitative or quantitative? |
|
Sensitivity |
9. How often is the test positive when a mutation is present? |
|
Specificity |
10. How often is the test negative when a mutation is not present? |
|
11. Is an internal QC program defined and externally monitored? |
||
12. Have repeated measurements been made on specimens? |
||
13. What is the within- and between-laboratory precision? |
||
14. If appropriate, how is confirmatory testing performed to resolve false-positive results in a timely manner? |
||
15. What range of patient specimens has been tested? |
||
16. How often does the test fail to give a useable result? |
||
17. How similar are results obtained in multiple laboratories using the same, or different, technology? |
||
Clinical Validity | Sensitivity |
18. How often is the test positive when the disorder is present? |
Specificity |
19. How often is the test negative when a disorder is not present? |
|
20. Are there methods to resolve clinical false-positive results in a timely manner? |
||
Prevalence |
21. What is the prevalence of the disorder in this setting? |
|
22. Has the test been adequately validated on all populations to which it may be offered? |
||
23. What are the positive and negative predictive values? |
||
24. What are the genotype/phenotype relationships? |
||
25. What are the genetic, environmental, or other modifiers? |
||
Clinical Utility | Intervention |
26. What is the natural history of the disorder? |
Intervention |
27. What is the impact of a positive (or negative) test on patient care? |
|
Intervention |
28. If applicable, are diagnostic tests available? |
|
Intervention |
29. Is there an effective remedy, acceptable action, or other measurable benefit? |
|
Intervention |
30. Is there general access to that remedy or action? |
Element | Component | Specific Question |
---|---|---|
31. Is the test being offered to a socially vulnerable population? |
||
Quality Assurance |
32. What quality assurance measures are in place? |
|
Pilot Trials |
33. What are the results of pilot trials? |
|
Health Risks |
34. What health risks can be identified for follow-up testing and/or intervention? |
|
35. What are the financial costs associated with testing? |
||
Economic |
36. What are the economic benefits associated with actions resulting from testing? |
|
Facilities |
37. What facilities/personnel are available or easily put in place? |
|
Education |
38. What educational materials have been developed and validated and which of these are available? |
|
39. Are there informed consent requirements? |
||
Monitoring |
40. What methods exist for long-term monitoring? |
|
41. What guidelines have been developed for evaluating program performance? |
||
ELSI | Impediments |
42. What is known about stigmatization, discrimination, privacy/confidentiality, and personal/family social issues? |
43. Are there legal issues regarding consent, ownership of data and/or samples, patents, licensing, proprietary testing, obligation to disclose, or reporting requirements? |
||
Safeguards |
44. What safeguards have been described and are these safeguards in place and effective? |
NOTE: ELSI = ethical, legal, and social implications; QC = quality control.
SOURCE: CDC, 2013.
The Evaluation of Genomic Application in Practice and Prevention Framework
CDC established the EGAPP initiative in 2004 to analyze the potential benefits and harms of genetic tests. The EGAPP Working Group (EWG), an independent panel, developed a systematic process for evidence-based assessment that focuses on genetic tests and other applications of genomic technology modeled on the criteria from ACCE and USPSTF.
The EGAPP method consists of a topic-selection process, an analytic framework with key questions to frame the evidence review, a systematic review of evidence, and recommendations based on the evidence. Once a topic is selected for review, EWG drafts an analytic framework (similar to those used by USPSTF) to illustrate explicitly the clinical scenario, the intermediate and long-term health outcomes of interest, and the key questions to be addressed. The analytic framework constitutes the clinical scenario and must be customized for each topic.
The first and over-arching key question is whether there is direct evidence that using the test leads to clinically meaningful improvement in outcomes or in medical or personal decision making. Direct, good-quality evidence of clinical utility that addresses specific measures of the outcomes of interest (e.g., from well-designed clinical trials) renders later questions unnecessary, but that has seldom been the case for genetic tests evaluated by EWG. Additional questions outline an indirect-evidence pathway to demonstrate clinical utility and, in more specific terms, address such issues as the following:
- How valid and reliable are available tests?
- How well will the tests predict outcomes?
- What actions should be based on results?
- What benefits and harms are associated with the clinical use of the tests?
- How should the medical community, public health, and policy makers respond?
The EGAPP method integrates knowledge and experience from existing processes, such as a systematic review process from ACCE; assessment of the quality of individual studies, the adequacy of overall evidence, the level of certainty, and the magnitude of net benefit from USPSTF; and contextual issues from GRADE.4 The method combines an analytic framework with an evidence-based assessment and allows customization according to clinical scenario (Teutsch et al., 2009; Sun et al., 2011; Morrison and Boudreau, 2012).
The Genetic Testing Evidence Tracking Tool
The committee also reviewed the Genetic testing Evidence Tracking Tool (GETT), developed by Rousseau and colleagues, which includes a list of 72 defined items and questions grouped into 10 categories and 26 subcategories to “fill in the gaps” of existing frameworks (Rousseau et al., 2010; see Table 3-3). The tool does not set priorities for the order of assessment other than first carefully defining the condition and ultimately identifying which decisions require further investigation. The detailed questions posed by the GETT are noted in Appendix B.
In effect, the GETT provides a structure for systematic identification and organization of published evidence. The main goal is to help stakeholders to determine whether the knowledge base is sufficient for assessing the health care benefits of a given molecular-genetic test and identifying specific research subjects that require greater emphasis. Factors considered include epidemiology and genetics of the condition, available diagnostic tools and their analytic and clinical performance, availability of quality-control programs, laboratory and clinical best-practice guidelines, clinical utility and effects on health and the health care system, the quality of the supporting data, and psychosocial, ethical, and legal implications. The objective is to provide a more detailed instrument by which those factors can be considered and that can be applied in a variety of contexts. In the clinical utility category, for example, the reviewer is asked to provide the documented benefits and risks and their frequency and severity. A major strength of the tool is the high resolution provided by the detailed 72 items or questions in the list, which allows one to identify specific subjects that need greater attention to develop a sufficient evidence base for decision making. The high resolution should also mitigate the all-or-none effect of approving or disapproving a test and would allow reviewers to decide which subjects they rank most important in decision making. As a proof of concept, the tool was applied to three diseases, which were selected because of their wide array of mutation characteristics: hemochromatosis, thrombophilia, and fragile-X syndrome. The authors emphasized the importance of assessing new proposed frameworks by applying different disease scenarios.
___________________
4 The GRADE—Grading of Recommendations Assessment, Development and Evaluation—working group began in 2000 and has developed an approach to grading quality (or certainty) of evidence and strength of recommendations. Many international organizations have provided input into the development of the GRADE approach, which is now considered the standard in guideline development (Guyatt et al., 2011). Available at: http://gradeworkinggroup.org (accessed January 31, 2016).
TABLE 3-3 Characteristics and Definitions of Themes and Subthemes of GETTa
1 Overview of the disease: epidemiology and genetics | 1 Disease prevalence 1.2 Disease outcomes 1.3 Clinical management and treatment 1.4 Costs associated with disease 1.5 Pattern of inheritance 1.6 Genetic heterogeneity 1.7 Mutation prevalence 1.8 Mutation penetrance 1.9 Neomutation rate |
2 Diagnostic tools | 2.1 Approaches other than molecular 2.1.1 Methods 2.1.2 Analytical validity 2.1.3 Clinical validity 2.1.4 Infrastructures and costs 2.2 Molecular approaches 2.2.1 Methods 2.2.2 Analytical validity 2.2.3 Clinical validity 2.2.4 Infrastructures and costs 2.2.5 Interpretation 2.2.6 Consensus or best practice guidelines |
3 Quality improvement program | 3.1 Internal 3.2 External |
4 Clinical utility | 4.1 Objectives |
5 Screening or diagnostic strategies | |
6 Impacts on the health care system | 6.1 Foreseeable needs for testing 6.2 Costs (including replacement of existing analyses, cost/effectiveness and cost/utility studies) 6.3 Tests accessibility 6.4 Availability and accessibility of professional services, health care and follow-up, expertise and training |
7 Psychological and social aspects of the analysis | |
8 Ethical and legal aspects of the analysis | |
9 Synthesis | |
10 Research priorities | |
11 References |
a See Appendix B for GETT detailed questions.
SOURCE: Rousseau et al., 2010. Reprinted from De Gruyter Clinical Chemistry and Laboratory Medicine, Walter De Gruyter GmbH Berlin Boston, 2010. Copyright and all rights reserved. Material from this publication has been used with the permission of Walter De Gruyter GmbH.
The McMaster University Evaluation Framework
The Ontario Provincial Advisory Committee on New Predictive Genetic Technologies commissioned an analysis by McMaster University to provide guidance for technology assessment and coverage decisions related to emerging genetic testing services in Canada (Giacomini et al., 2003). The analysis focused not only on decisions that are an obvious “no” or “yes” but on “gray zones” in which evaluation is uncertain: those with unclear intended purpose; poorly defined standards of effectiveness, efficiency, and other evaluative criteria to merit coverage; underdeveloped performance standards; or absent, ambiguous, or incomplete information (Giacomini et al., 2003).
The authors of the framework defined the general criteria for evaluation of health technologies and outlined them; Table 3-4 distills basic questions of effectiveness, efficiency, normative issues, and technologic assembly.
The McMaster evaluation model covers three domains for decision makers to consider: evaluation criteria, acceptable cutoffs, and conditions on coverage (see Figure 3-1).
Evaluation Criteria
In their review of the literature, the authors summarized issues germane to genetic tests according to numerous advisory bodies and distilled six evaluation criteria that apply to health-technology assessment: the intended purpose of the test, the effectiveness of the test compared with other approaches in accomplishing its purpose, additional effects beyond the intended purpose, the aggregate costs of using the test, the demand for use of the test, and the cost-effectiveness of the test relative to that of other covered services that have the same purpose. The authors note that the description and evaluation of the purpose of the test should precede discussion of other criteria; if the purpose of the test is not deemed “worthwhile” (a value judgment), it “should neither be covered nor evaluated further.”
Acceptable Cutoffs
For each criterion established above, there must be standards that govern decision making so that evaluation will be clear and consistent. Giacomini and colleagues explicitly noted the “negotiability” of the standards and the gray zones that might exist in attempting to operationalize the decision-making process. They suggest that cutoffs “could be derived deductively and in the abstract, in the absence of a given coverage case” through application of normative principles that extend beyond the evaluation framework itself—for example, cost-effectiveness ratios based on established acceptable cost per life-year gained—while noting the difficulty of this approach. Alternatively, cutoffs could be based on existing precedents regarding the decisions already made about similar tests; such an approach “requires good institutional memory, not only of the decisions made in the past but also of the reasons for making them”; this evokes the need for some type of structured repository of prior decisions. Finally, cutoffs could be determined by comparison with those of other “technologies already covered and well-accepted in the health system” (Giacomini et al., 2003). Thus, for any given criterion listed above, the test in question can be compared with other covered services that accomplish the same goal.
TABLE 3-4 McMaster University General Criteria for the Evaluation of Health Technologies
Effectiveness and Efficiency Questions | |
---|---|
Does the technology work? | |
Is empirical evidence available regarding the technology’s effectiveness? | |
How well does evidence of effectiveness comply with clinical epidemiology principles for critical appraisal? | |
Does the technology work well enough? Compared to what? | |
Is it cost effective? | |
Is the technology cost-effective? Compared to what? For what purpose? | |
How much does the technology cost, and how effective is it? | |
What is the distribution of its costs and benefits across members of society? Who pays the costs? Who enjoys the benefits? | |
Normative Questions | |
Do individuals want it? | |
How important are personal preferences and principles of autonomy in valuing the technology? | |
What economics and institutions influence these personal preferences (e.g., marketing, culture, clinicians)? | |
What social relationships influence personal preferences (e.g., relatives with a stake in the genetic information)? | |
Does the community want it on behalf of individuals? | |
How important are principles of solidarity, compassion, etc., in valuing the technology? | |
Does the community have a direct interest in offering this technology to individuals (i.e., due to externalities)? | |
Have individuals been granted legal entitlements or rights to this technology (i.e., by legal precedent or legislation)? | |
Is it equitable to cover this technology at the expense of other things? | |
Are the costs and benefits of coverage shared fairly (not equally, but equitably) across members of society? | |
Is the technology otherwise ethical? | |
Many other ethical principles may come into play, e.g., human rights, dignity, reproductive rights, discrimination, privacy, and so forth. | |
Assembly Questions | |
What is the technology and what is it for? | |
Why address this technology (e.g., “new” genetic tests) as distinct from other health technologies (e.g., conventional genetic diagnostics)? | |
What features define this technology and its subtypes? | |
Should the technology be defined narrowly (e.g., lab test) or broadly (e.g., all necessary services to accomplish a clinical aim)? | |
Is its purpose to produce well-being, health, mental health, physical health, knowledge, or something else? | |
What are the technology’s potential effects besides its intended purposes? | |
How is it situated? | |
Which other technologies does this technology entail (e.g., interventions subsequent to diagnosis, genetic counseling, etc.)? | |
Which existing technologies does this technology displace or otherwise affect? | |
What are this technology’s alternatives (as suggested by its various purposes, above)? |
Whose is it and who is it for? | |
Which stakeholders are interested in this technology—for benefit, for profit, for information? | |
What are the political, economic, social relations between these stakeholders? | |
For which subpopulations can the technology currently work well? | |
Ideally, for which subpopulations should such technology work well? |
SOURCE: Giacomini, M., F. Miller, and G. Browman. 2003. Confronting the “gray zones” of technology assessment: Evaluating genetic testing services for public insurance coverage in Canada. International Journal of Technology Assessment in Health Care 19(2):301-316. Reproduced with permission.
Conditions on Coverage
Giacomini and colleagues (2003) note that decisions about the use of genetic tests need not always be strictly binary and that there might be gray zones in which coverage decisions could be made conditionally so that promising new tests could be covered in some contexts. That concedes the importance of dealing with inexact evaluations, which are often encountered in connection with rapidly evolving technologies. The coverage conditions include clarification of purpose; improved research protocols; periodic re-evaluation of evidence; enhanced interventions into personal, family, and societal effects; published clinical-practice protocols and guidelines; ethics protocols; legal regulation; and priority setting (weighing the value of different health services).
Thus, the McMaster University evaluation framework provides a thorough approach and rich detail for making decisions about genetic testing. The three domains of the McMaster evaluation framework (establishing evaluation criteria, determining acceptable cutoffs for each criterion, and determining conditions of coverage for gray zones) provide the foundation of the model, whereas the “effectiveness and efficiency,” “normative,” and “assembly” questions help to fill in the framework. The “normative” questions provide consideration of personal preferences and autonomy, societal preferences, societal equity, and the balance of various influences (marketing, culture, clinicians, family members, and so on). Assessment of the gray zones of decision making requires sensitive, multifaceted instruments, such as this framework provides.
The Frueh and Quinn Framework
Another evaluative framework considered by the committee was proposed by Frueh and Quinn (2014). It focuses on the reimbursement perspective and provides examples drawn from the companion diagnostic biomarker tests, but it includes references to other types of genetic tests. The authors suggest that analytic validity, clinical validity, and clinical utility “offer too little guidance to structure a rational and predictable interaction between the test developer and the payer or technology assessment body.” They identify three axes that describe considerations that might be taken into account during a technology assessment.
The first axis represents functional categories of genetic tests, that is, their purpose in a clinical setting. The authors identify six common categories of clinical tests (not limited to genetic testing):
- risk assessment
- screening of asymptomatic people
- diagnostic tests in response to symptoms
- treatment selection
- monitoring of treatment effects
- tests that serve as outcome measures
The second axis identified by Frueh and Quinn is the test’s value proposition, which is necessarily a comparative endeavor. In this category, they list seven common value propositions:
- Measure the same analyte but faster or less expensively.
- Measure the same analyte but with higher accuracy.
- Measure a target that is entirely new.
- Generate a more accurate prognosis.
- Resolve a previously ambiguous test with a higher-level test.
- Provide a diagnosis where all other methods have failed.
- Rule out patients for further tests or procedures.
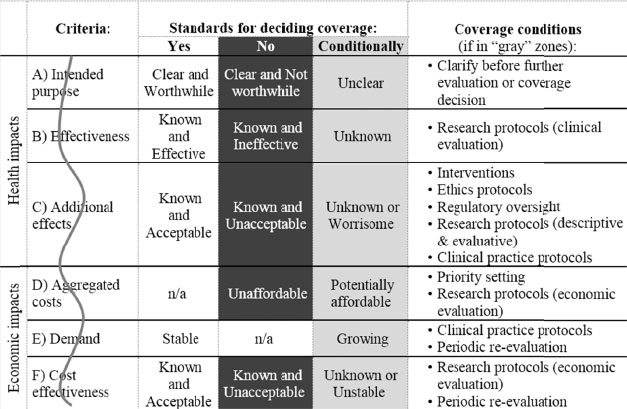
NOTE: The vertical wavy line represents the “jagged cutoffs between yes/no coverage decisions for all of the evaluation criteria outlined in Figure 3-1. The jaggedness represents the negotiability of those lines. To apply an evaluative criterion in an accountable and consistent way, decision makers require a clear delineation of what is “good enough” from what is not. This value judgment is the crucial analytic step to make each criterion operational. The unit of analysis for this evaluative task is the decision criterion (not the genetic testing service)” (Giacomini et al., 2003).
SOURCE: Giacomini, M., F. Miller, and G. Browman, 2003. Confronting the “gray zones” of technology assessment: Evaluating genetic testing services for public insurance coverage in Canada. International Journal of Technology Assessment in Health Care 19(2):301-316. Reproduced with permission.
The third axis represents outcome metrics that characterize the use of the test in clinical practice. Again, they list a number of common outcome metrics that can be considered (but note that many others are possible):
- increased survival
- increased progression-free survival
- increased quality of life
- decreased pain
- value of knowing a diagnosis
- ability to make childbearing decisions
The authors suggest that tests that exist in different categories in the three axes will be evaluated differently. In their examples, new screening tests might “require a very high level of confidence regarding their effects on large populations who are not at a priori risk, but who will be exposed to anxiety and more invasive and definitive tests”; at the same time, if a screening test is already in wide use, a new method that can accomplish the screening might be evaluated primarily on the basis of its accuracy and cost compared with the gold standard. Frueh and Quinn raise the question, “How . . . can we help guide developers, dossier authors, and technology assessors—with more granularity than the clinical validity–clinical utility scheme, but without requiring dozens of guidance documents?” To accomplish that, the authors propose a set of six questions to guide assessment of genetic tests:
- Who should be tested and under what circumstances?
- What does the test tell us, that we did not know without it?
- Does the outcome change in a way we find value in, relative to the outcome(s) obtained without the test?
- Can we act on the information provided by the test?
- Will we act on the information provided by the test?
- If the test is to be employed, can we afford it?
Those questions address the major themes identified in the three axes listed above, often combining aspects of them within the same question or set of questions. The authors also address the issue of uncertainty and the importance of distinguishing “bona fide areas of uncertainty and concern” from ones “that are merely conjectural.” In many cases, they argue, “discussing these uncertainties explicitly has the potential to increase agreement, or at least, cast sharper light on specific areas of disagreement among test developers, clinician advocates, regulators, and payers.” They conclude that addressing communication gaps is necessary to avoid the situation in which payers (and presumably other test assessors) “may finish reviewing the data with a sense of uncertainty, perceiving the data as inadequate, confusing or riddled with evidence gaps, while test developers may complain equally nonspecifically that payers’ standards are ‘too high.’”
COMPARATIVE ANALYSIS OF EVALUATION METHODS
The committee considered the similarities and differences between the various methods in purpose, approach, strengths, and weaknesses. Of the evaluation methods reviewed, the USPSTF method and Fryback–Thornbury hierarchy were developed for general health-
technology assessment and were not designed specifically for genetic tests. The USPSTF method is a specific use-case for health care technology: the evaluation of preventive services being performed in the general population. The evaluation criteria are focused on high-level clinical-utility outcomes (morbidity and mortality) that might not apply in all clinical scenarios. In contrast, the Fryback–Thornbury hierarchy recognizes the clinical value of diagnostic efficacy and diagnostic thinking. These outcomes are highly relevant in the context of genetic diagnostic testing, in which the intended use of the test is to aid in refining the differential diagnosis or to establish a specific molecular diagnosis. The frameworks proposed by Giacomini et al. (2003) at McMaster University and Frueh and Quinn (2014) are intended to be used by payers and other stakeholders who are considering whether to use or cover the cost of genetic tests.
Each of the evaluation methods identifies a “topic” implicitly or explicitly. The process involves defining the clinical scenario, the test, and the patient population being tested. In the McMaster University evaluation framework, the first criterion is whether the intended purpose of the test is “clear and worthwhile.” That immediately creates a value judgment on the part of the evaluator: the clinical indication for the test might be clear—for example, to establish a diagnosis in a symptomatic person, to provide information about carrier status for recessive disorders, to conduct prenatal screening for genetic abnormalities in a fetus, to provide predictive information about a person’s future health status—but the decision about whether a particular use of the test is “worthwhile” depends on the stakeholder’s perspective. Giacomini and colleagues suggest that “services with a worthwhile purpose merit further evaluation and consideration for coverage. Services with a purpose deemed not-worthwhile should neither be covered nor evaluated further.” Articulation of the intended use of a test is also included in Frueh and Quinn’s three axes and specified in their first question: “Who should be tested and under what circumstances?” The issue of whether a test has value is also directly addressed by Frueh and Quinn: “Does the outcome change in a way we find value in, relative to the outcome(s) obtained without the test?” In the USPSTF method, the purpose of testing is defined as preventive; in the EGAPP method, different outcomes of interest can be evaluated. In each of those cases, an analytic framework is developed to evaluate key questions that are specific to the topic through formal evidence reviews. ACCE and GETT each define comprehensive lists of questions that are specific to genetic testing scenarios, but neither defines whether a particular use case is worthwhile. Similarly, the Fryback–Thornbury hierarchy can be applied broadly to any health technology and does not require a value judgment; however, the hierarchic levels of efficacy broadly reflect different intended purposes of any health technology and can thus be mapped to different outcomes of interest that reflect the purposes of the genetic test.
Evidence evaluation is also handled differently by the various methods. The Frybeck–Thornbury hierarchy does not define evidence criteria, but it recognizes that some types of evidence (such as that from RCTs) are preferable for particular clinical situations. Furthermore, the hierarchic nature of the evaluation is such that failure to meet the standard at a lower level (e.g., “technical efficacy”) renders assessments at the higher levels unnecessary and thereby greatly limits the scope and resources required to determine efficacy in some cases. Only the USPSTF and EGAPP methods define a specific analytic framework that focuses on key questions that are designed to address the outcome of interest. Those methods also define criteria for describing the strength of evidence, which is included in the final high-level recommendations regarding use of the genetic test. In many cases, evidence is insufficient to support a recommendation. The McMaster University evaluation framework makes special note of the gray zones that might occur in examining particular criteria and addresses the problem of
insufficient evidence in such rapidly evolving fields as genetics. In this process, conditional coverage decisions might be considered, depending on the clinical context. Similarly, Frueh and Quinn highlight the comparative nature of health-technology assessment, stating that “analysis and debate should focus on the comparator test(s) or outcome(s), the units of measure for the improvement, and the factors that create uncertainty about the outcome.”
Many of the methods include an economic assessment. The final question articulated by Frueh and Quinn is, “If the test is to be employed, can we afford it?” In the ACCE framework, financial costs and economic benefits are considered in the category of clinical utility; in the Fryback–Thornbury hierarchy, cost–benefit analysis and cost-effectiveness are considered at the level of societal efficacy, although these criteria are presented in general terms that do not reach the level of detail provided by some of the other models. Cost assessment is a major component of GETT and considered in the category of “impacts on the health care system,” including the potential for carrying out detailed cost-effectiveness and cost-utility analyses. Considerations from the payer perspective are more strongly emphasized in the McMaster University evaluation framework than in other models, with detailed questions of cost-effectiveness from a variety of stakeholder perspectives, probably because of its proposed primary use for payer decision making in the Canadian national health care system. The McMaster University evaluation framework directly addresses coverage decisions, and three of the six criteria that it articulates (aggregate costs per patient, demand for testing, and cost-effectiveness) are related to economic factors that a payer must consider. Its framework also recommends “coverage with evidence collection” in some circumstances.
Although some of the evaluation methods were developed specifically for genetic testing, most were established during the era of single-gene testing before the emergence of next generation sequencing (NGS) and the ability to test hundreds or thousands of genes simultaneously. For example, clinical whole-exome sequencing is most often applied in challenging cases that have multiple clinical features and unclear diagnoses (Biesecker and Green, 2014). It is therefore difficult to answer some questions outlined by ACCE and GETT—such as those related to the specific clinical disorder to be studied, the clinical performance of the test according to the target population, genetic heterogeneity, a new mutation rate, mutation prevalence, penetrance, and the prevalence or the natural history of the disorder—because the answers differ gene by gene, and some will be established only after the test result is known.
The methods reviewed share some characteristics in the criteria used for evaluation of health technology. The four domains map broadly to the ACCE criteria, with the Fryback–Thornbury hierarchy representing clinical utility in three categories—patient-outcome efficacy, therapeutic efficacy, and diagnostic-thinking efficacy. The USPSTF method represents a specific use case for evaluating health care interventions in the context of preventive services in the general population and thus emphasizes patient outcomes, such as morbidity and mortality, as high-level end points. EGAPP organizes evidence into the ACCE categories and evaluates the chain of evidence by using a framework similar to USPSTF. The McMaster University evaluation framework identifies six criteria, one of which (effectiveness) depends on the intended purpose of the test; it also introduces consideration of aggregate costs, use metrics, and cost-effectiveness criteria, which are important from the health care system perspective. GETT, although not explicitly intended as an evaluation method, provides a systematic model for organizing published evidence in 10 main categories.
Table 3-5 provides a comparison of the frameworks with regard to purpose, approach, strengths, and weaknesses.
INTEGRATION BETWEEN GENETIC TEST ASSESSMENT METHODS AND RELEVANT OUTCOMES
As indicated in the McMaster University evaluation framework, the first step of an evaluation is to determine whether the purpose of genetic testing in a particular clinical scenario is clear and worthwhile. That concept is also the subject of two questions posed by Frueh and Quinn. Thus, stakeholders who evaluate a genetic test need to link the purpose of the test with the desired outcome of testing. If the evaluator deems the purpose to have intrinsic value, the evaluation should be targeted to the appropriate type, amount, and quality of evidence required to make a decision about a particular genetic testing topic. Decisions about coverage of a particular genetic test will necessarily require comparison with other tests regarding economic factors—such as aggregate costs per patient, demand for testing and volume of test requests, and cost-effectiveness—all of which are related to the decision about whether the purpose of the test (and therefore the anticipated outcome) is worthwhile.
The EGAPP working group previously outlined a broad set of clinically relevant outcomes that could be considered in the evaluation of genetic tests (Botkin et al., 2010), so the committee sought to understand how those outcomes could be mapped to the genetic test assessment methods described above. In that regard, the Fryback–Thornbury hierarchy proved to be a useful construct because it provided a number of categories that had clear parallels to evidence types in the ACCE criteria (see Table 3-2). However, one aspect of genetic information that is not directly addressed is personal utility, that is, information that might or might not be medically actionable but could have meaning and value to the individual person. The committee added the concept of personal utility to the hierarchy at a level that complements the physician’s diagnostic efficacy. Table 3-6 details the modified Fryback–Thornbury hierarchy for genetic testing, compares the levels of the hierarchy with the ACCE criteria, and maps relevant outcomes previously outlined by EGAPP.
The most basic outcomes of genetic testing are the technical aspects that are related to the ability of a test to detect relevant genetic variation, which is equivalent to Fryback–Thornbury’s “technical efficacy.” Analytic validity involves accurate data generation and validation of the performance of a test against other gold-standard tests (if any). Although no medical test is perfect, the degree of accuracy and the tolerance for false-negative and false-positive analytic results might differ, depending on the clinical scenario.
Layered over the analytic performance of a test are its clinical sensitivity and specificity, including the interpretation of the clinical significance of variants (pathogenic, uncertain, or benign) and case-level assessment of results. Clinical sensitivity (the proportion of affected people who test positive) and clinical specificity (the proportion of unaffected people who test positive—false-positive results) can be measured directly when gold-standard clinical diagnostic criteria are available. However, in many clinical scenarios in which genetic testing might be considered as a means of establishing a definitive diagnosis or defining future risk of disease, the true positives in the population being tested are not known. In that scenario, the diagnostic yield of genetic testing approximates clinical sensitivity, but the actual numbers of true positives, false positives, false negatives, and true negatives will remain unknown. Those outcomes depend heavily on the population being tested because of the differences in disease prevalence, mutation frequency, mutation spectrum, and the appearance of clinical features over time. False-positive and false-negative results can have a detrimental effect on patients, and the predicted frequency of such events must be considered (Hunink et al., 2014).
TABLE 3-5 Comparison of Frameworks
Method | Purpose | Approach | Strengths | Weaknesses |
---|---|---|---|---|
USPSTF | Preventive interventions in primary care | Formal analytic framework; evidence assessment related to key questions to establish a “chain of evidence†| Formal grading system incorporating evaluation of benefits and harms and rating of evidence | Focus on preventive services results in a focus on clinical-utility outcomes that are not relevant to all clinical applications |
Fryback–Thornbury | General medical-test assessment | Hierarchic representation of levels of efficacy for medical tests | Allows an evaluator to determine what evidence types need to be assessed for a given test purpose or desired outcome | Lacks a formal evidence-based assessment procedure |
ACCE | Analytic process for evaluating evidence on genetic tests | Standard set of 44 questions that are organized according to different evidence types (analytic validity, clinical validity, clinical utility, ELSI) | Provides a highly granular approach to assessing different evidence types | Does not provide details on evaluating the strength of evidence; developed for single-gene tests and may be difficult to extend to multigene panels or genome-scale sequencing tests |
EGAPP | Systematic approach to evidence-based assessment of genetic tests | Hybrid approach using analytic framework similar to USPSTF and evaluation of evidence types articulated by ACCE | Flexibility to evaluate different “topics†in genetic testing, including a wide array of potential outcomes of interest, and integration of formal evidence-based reviews | Focus on single-gene tests may be difficult to extend to broader genomic technologies |
GETT | Structure for systematic identification and organization of published evidence on genetic testing | List of 72 defined items grouped into categories | Helps stakeholders to determine whether the knowledge base is sufficient for genetic-technology assessment | Does not provide details on evaluating the strength of evidence; developed for single-gene tests and may be difficult to extend to multigene panels or genome-scale sequencing tests |
McMaster University | Evaluation model to guide public coverage for new predictive genetic tests in Ontario, Canada | Combines technology assessment with coverage decision making from payer’s perspective | Defines criteria for determining coverage, anticipates the need for payers to identify evidentiary thresholds, and considers conditional coverage scenarios | Developed for the Canadian health system; lacks a formal evidence-based assessment procedure |
Method | Purpose | Approach | Strengths | Weaknesses |
---|---|---|---|---|
Frueh and Quinn | Framework to facilitate communication between test developers and health-technology evaluators | Relevant to both regulatory and payer decisions | Articulates several axes of testing that provide more nuanced or diverse evaluation outcomes than traditional clinical validity and clinical utility | Lacks a formal evidence-based assessment procedure; examples of applications of six questions directed mainly toward companion diagnostics |
NOTE: ACCE = analytic validity, clinical validity, clinical utility, and associated ethical, legal, and social implications; EGAPP = Evaluation of Genomic Application in Practice and Prevention; ELSI = ethical, legal, and social implications; GETT = Genetic testing Evidence Tracking Tool.
In the Fryback–Thornbury hierarchy, diagnostic-thinking efficacy (the ability of a clinician to arrive at a diagnosis) depends on clinical validity. As a result of accurate diagnosis, a clinician can provide improved information about the natural course of a condition and stop the pursuit of potentially expensive and invasive diagnostic tests, often referred to as the diagnostic odyssey. Improving the efficacy of diagnosis is also of interest to payers (Gross et al., 2008), and new genetic tests that interrogate hundreds or thousands of genes simultaneously could offer comparative advantages in arriving at a diagnosis earlier in the disease course. Clinical validity depends on a robust association between the gene and the disease or condition and on understanding the natural history of the disease and the relative and absolute risks conferred by the genetic variant.
In addition to direct effects on medical care, genetic information can provide a greater sense of control and an ability to act and develop new supports and treatments that can have a favorable effect on patient outcomes. Furthermore, genetic information can affect the family as well as the patient, multiplying the benefits and harms. Sharing genetic information within families can affect family dynamics favorably or adversely, as well as affecting the health of the family.
Genetic testing can increase the precision and accuracy of diagnosis and thus affect clinicians and their clinical management decisions directly. For example, genetic testing can differentiate types of long-QT syndrome, which can be indistinguishable in electrocardiography, clinical symptoms, and family history (Napolitano et al., 2015). Identification of the molecular type has treatment implications with respect to triggers to avoid arrhythmias and provide maximally beneficial medications. Identification of a mutation within the family allows an efficient, effective method for identifying other at-risk family members for long-QT syndrome. Once a precise diagnosis is established, more targeted and efficacious prevention, surveillance, and treatment can be established, and ineffective treatments that waste resources or can be associated with adverse outcomes can be avoided. If patients are convinced that a treatment is the correct treatment, they might be more likely to comply with the therapeutic plan (Horne et al., 2013). For many genetic conditions, there are established management guidelines that make up a standard of care. Establishing a definitive genetic diagnosis can thus enable a clinician to establish and adhere to appropriate management plans and achieve therapeutic and management efficacy. A definitive diagnosis can allow a patient to avoid unnecessary procedures or medications. In some cases, defined clinical benefits that result in improved outcomes at the level of morbidity and mortality can be demonstrated.
At the societal level, effective genetic testing should have a favorable effect on health and allow effective health interventions throughout an entire population. However, it could adversely
affect health disparities (Hall and Olopade, 2005) and the cost of health care (Phillips et al., 2014). Depending on how genetic information is used and managed and how members of society react to the information, it could improve public perception of genetics or raise concerns about genetic determinism, tolerance for genetic differences, and discrimination or intentional selection against “undesirable” traits or selection for “desirable” traits. It is important to examine ELSI and the effects of applying genetic technologies on a large scale (Clayton, 2003).
SUMMARY
The committee reviewed methods that have been proposed for reviewing evidence and making decisions about using medical tests, including several methods designed specifically for genetic tests. The committee also compared those methods and offers a synthesis that maps clinically relevant outcomes of interest to a hierarchic evidence structure.
Many of the genetic test assessment methods cover the three common domains of evaluation: analytic validity, clinical validity, and clinical utility. The ACCE and Fryback–Thornbury models include an additional domain: societal effects. Some evaluation frameworks (such as the Fryback–Thornbury hierarchy) provide general conceptual guidance, and analytic frameworks (such as USPSTF and EGAPP) provide additional detail for important questions regarding the relevant populations, interventions, comparators, outcomes, time points, and settings.
The McMaster University evaluation framework provides a thorough approach and rich detail for making decisions about genetic testing. The three domains of the McMaster evaluation framework—establishing evaluation criteria, determining acceptable cutoffs for each criterion, and determining conditions of coverage for gray zones—provide the foundation of the model, whereas the “effectiveness and efficiency,” “normative,” and “assembly” questions help to fill in the evaluation framework. The “normative” questions provide consideration of personal preferences and autonomy, societal preferences, societal equity, and the balance of various influences (marketing, culture, clinicians, family members, and so on). Finally, the assessment of the gray zones of decision making requires sensitive, multifaceted instruments, which this framework provides.
The four domains map broadly to the ACCE criteria, with the Fryback–Thornbury hierarchy representing clinical utility in three categories: patient-outcome efficacy, therapeutic efficacy, and diagnostic-thinking efficacy. The USPSTF method represents a specific use case for evaluating health care interventions in the context of preventive services in the general population and thus emphasizes patient outcomes, such as morbidity and mortality, as high-level end points. EGAPP organizes evidence into the ACCE categories and evaluates the chain of evidence by using a framework similar to USPSTF. The McMaster University evaluation framework identifies six criteria, one of which (effectiveness) depends on the intended purpose of a test; it also introduces consideration of aggregate costs, use metrics, and cost-effectiveness criteria that are important from the health care system perspective. GETT, although not explicitly intended as an evaluation method, provides a systematic model for organizing published evidence in 10 main categories; its evaluation process, the first step of which is to determine whether the purpose of genetic testing in a particular clinical scenario is clear and worthwhile, is also the subject of two questions posed by Frueh and Quinn.
TABLE 3-6 Modified Fryback–Thornbury Hierarchic Model of Efficacy and Relevant Outcomes
Domain | Relevant Outcomes | Description | ACCE Comparison |
---|---|---|---|
Societal efficacy | Effect on health disparities Cost of health care Population-health intervention Perceptions of disabilities, eugenics Perspectives of genetic determinism |
Do cost–benefit or cost-effectiveness analyses indicate that the test has efficacy at the health system or societal level? Is there evidence of adverse societal effects directly attributed to the test? | ELSI |
Patient-outcome efficacy | Morbidity Mortality Other clinical end points (hospitalizations, procedures) Quality of life Options for prevention or therapy Ability to avoid adverse outcomes of ineffective treatments Options for reproductive planning Improved ability to plan for future events |
Do patients who undergo the test fare better than similar patients who do not? Do patients benefit from the use of the test? |
Clinical utility |
Therapeutic and management efficacy | Adherence to therapeutic regimen Planning surveillance, prevention, or treatment plans Targeted treatment or avoiding harms of treatment |
Does the test aid in planning treatment? Does the test change or cancel planned treatments? |
|
Diagnostic-thinking efficacy | Ending diagnostic odyssey and preventing expensive or invasive diagnostic tests Improved accuracy of prognosis |
Does the test help a clinician to come to a diagnosis? Does the test change a clinician’s pretest estimate of the probability of a specific disease? |
Clinical validity |
Diagnostic accuracy | Accurate molecular diagnosis Clinical sensitivity and specificity | Does the test result distinguish patients with and without the target disorder among patients in whom it is clinically reasonable to suspect that the disease is present? | |
Technical efficacy | Accurate detection of genetic variants Analytic sensitivity and specificity |
In the laboratory setting, does the test measure what it purports to measure accurately and reliably for indicated specimen types? | Analytic validity |
NOTE: ELSI = ethical, legal, and social implications.
Stakeholders who evaluate genetic tests need to link the purpose of a genetic test with its desired outcome. If the evaluator deems the purpose to have intrinsic value, evaluation of evidence should be targeted to the appropriate type of evidence and to the amount and quality of evidence required to make a decision about a particular genetic testing topic. Decisions about coverage of a particular genetic test will necessarily require consideration of economic factors—such as aggregate costs per patient, demand for testing and volume of test requests, and cost effectiveness—compared with those of other diagnostic modalities. All those factors are related to the decision about whether the purpose of the test in question, and therefore the anticipated outcome, are worthwhile.
The committee considered the integration between genetic test assessment methods and relevant outcomes, noting that stakeholders who evaluate genetic tests need to link the purpose of a test with its desired outcome. The committee has added the “efficacy” of personal utility to its modified Fryback–Thornbury hierarchy, that is, information that might not be medically actionable but could have meaning to the individual patient. Different stakeholders likely have different perspectives and issues that are important to them, but it is important to develop and use a framework that can be applicable in different testing scenarios.